Detecting Fake Product Reviews Using AI.
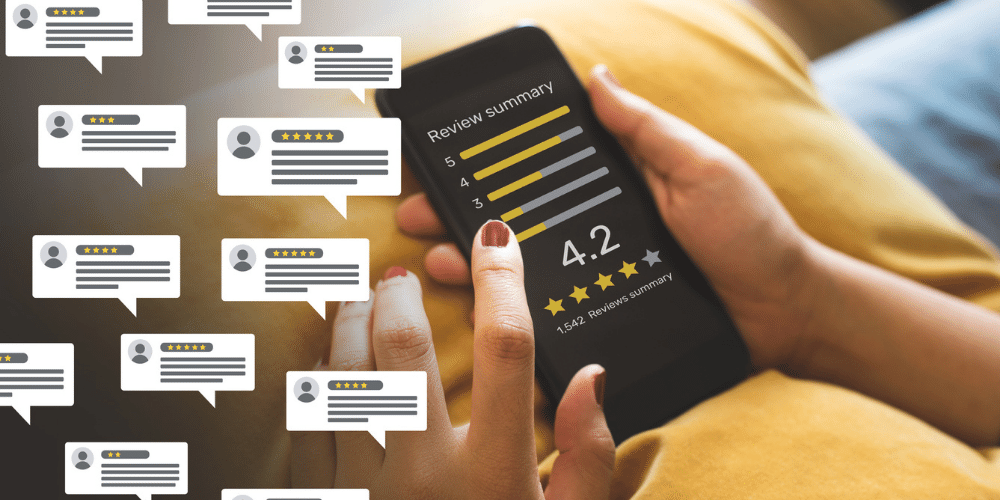
Reviews: a very important aspect these days, of feedback given to business owners by their customers. In a world full of online shopping and providing services, reviews act like a guiding light for customers. Customers write reviews to share their experience, to help other customers make a decision whether to buy a particular product and to reward a company for good product or good performance. Reviews help businesses, and good reviews help in getting more business, while bad reviews hurt the credibility of businesses.
Reviews being very effective in determining the sale of product can be faked to garner more sales and profits. Fake reviews can be written by people to manipulate a brand’s reputation or harm a competitor. They are usually written by non-customers and may involve financial transactions. It’s a common practice for e-commerce sites to solicit reviews from their customers, and many sites post reviews.
Fake reviews challenge the entire customer feedback system and violate the integrity of the e-commerce platform. Techniques for sorting out inappropriate reviews are therefore a step in the right direction. Identifying fake reviews is what self-learning artificial intelligence learns a lot.
AI proves to be very helpful in detecting a fake review. One way this can be done is by training a machine learning model on a large dataset of real and fake reviews, then using the trained model to predict whether a new review is fake or not. This can be done by looking for specific patterns or traits in the language used in reviews that are commonly found in fake reviews. For example, fake reviews often contain exaggerated praise or criticism, and may use excessive punctuation and exclamation marks. The model can also take into account other factors such as the source of the review and the reputation of the reviewer.
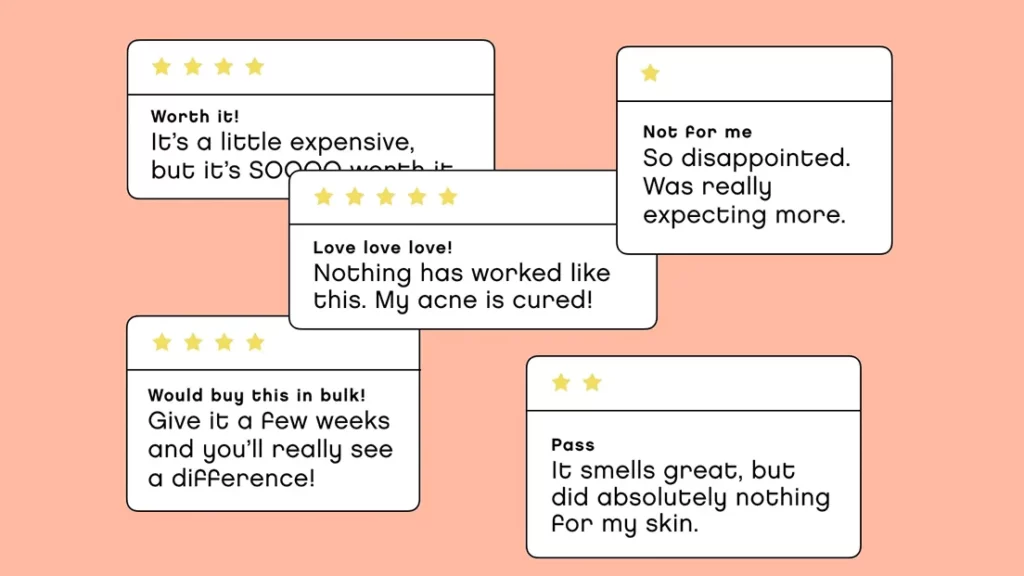
One approach is to use natural language processing (NLP) techniques to analyze the review text and look for clues that indicate it is fake. For example, fake reviews often use overly positive or repetitive language, and may include unnatural product or brand names.
Another approach is to use network analysis to look for patterns in how reviews and reviewers connect. Fake reviews are often written by people who are related to the product or brand being reviewed in a different way than genuine reviews.
Fake reviews are smart, but artificial intelligence is smarter. These systems will become increasingly difficult to deceive and will pose serious problems for fake reviewers in the future. To develop a new tool to recognize AI-generated text, researchers at Harvard University developed the MIT-IBM Watson AI Lab collaboration called the Giant Language Model Test Room (GLTR), the tool takes advantage of the fact that AI text generators use fairly predictable statistical patterns in text. Essentially, AI tools can determine if the text is too predictable and not human-written. Detects nearly three-quarters of fake reviews under test conditions compared to half of human reviewers.
Fake reviews do more harm than good. Feedback that does not reflect the actual customer experience only disrupts the relationship between brands and customers. Therefore, removing fake reviews can help businesses better assess their customer relationships. For example, self-learning systems have shown a better understanding of industry and product sentiment. The technology easily filters millions of reviews and continuously analyzes the entire market to remove fake reviews as well as present an unbiased representation of market sentiment towards a particular brand.
In this way, AI can help consumer-centric businesses better understand how their customers feel about their brands. Taking fake reviews out of this equation helps clarify what customers really think of your brand, rather than the falsely generated ones of certain outside influencers.
Overall, using AI to detect fake product reviews is an effective way to improve the credibility of online reviews and help consumers make more informed purchasing decisions.